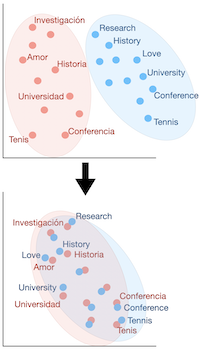
We consider the problem of aligning two sets of continuous word representations, corresponding to languages, to a common space in order to infer a bilingual lexicon. It was recently shown that it is possible to infer such lexicon, without using any parallel data, by aligning word embeddings trained on monolingual data. Such line of work is called unsupervised bilingual induction. By wondering whether it was possible to gain experience in the progressive learning of several languages, we asked ourselves to what extent we could integrate the knowledge of a given set of languages when learning a new one, without having parallel data for the latter. In other words, while keeping the core problem of unsupervised learning in the latest step, we allowed the access to other corpora of idioms, hence the name semi-supervised. This led us to propose a novel formulation, considering the lexicon induction as a ranking problem for which we used recent tools of this machine learning field. Our experiments on standard benchmarks, inferring dictionary from English to more than 20 languages, show that our approach consistently outperforms existing state of the art benchmark. In addition, we deduce from this new scenario several relevant conclusions allowing a better understanding of the alignment phenomenon.